
BOOKS - PROGRAMMING - Machine Learning for Engineers

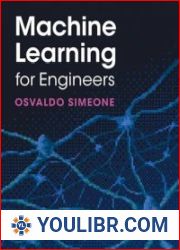
US $7.58

482928

482928
Machine Learning for Engineers
Author: Osvaldo Simeone
Year: 2023
Number of pages: 602
Format: PDF
File size: 17.0 MB
Language: ENG
Year: 2023
Number of pages: 602
Format: PDF
File size: 17.0 MB
Language: ENG
This self-contained introduction to Machine Learning, designed from the start with engineers in mind, will equip students with everything they need to start applying Machine Learning principles and algorithms to real-world engineering problems. With a consistent emphasis on the connections between estimation, detection, information theory, and optimization, it includes: an accessible overview of the relationships betweenMachine Learning and signal processing, providing a solid foundation for further study; clear explanations of the differences between state-of-the-art techniques and more classical methods, equipping students with all the understanding they need to make informed technique choices; demonstration of the links between information-theoretical concepts and their practical engineering relevance; reproducible examples using MATLAB, enabling hands-on student experimentation. Assuming only a basic understanding of probability and linear algebra, and accompanied by lecture slides and solutions for instructors, this is the ideal introduction to Machine Learning for engineering students of all disciplines. Advances in Machine Learning and Artificial Intelligence (AI) have made available new tools that are revolutionizing science, engineering, and society at large. Modern Machine Learning techniques build on conceptual and mathematical ideas from stochastic optimization, linear algebra, signal processing, Bayesian inference, as well as information theory and statistical learning theory. Students and researchers working in different fields of engineering are now expected to have a general grasp of Machine Learning principles and algorithms, and to be able to assess the relative relevance of available design solutions spanning the space between model-and data-based methodologies. This book is written with this audience in mind.
