
BOOKS - Amazon SageMaker Best Practices: Proven tips and tricks to build successful m...

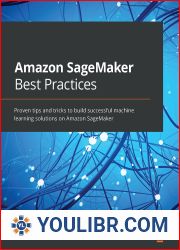
US $7.96

187429

187429
Amazon SageMaker Best Practices: Proven tips and tricks to build successful machine learning solutions on Amazon SageMaker
Author: Sireesha Muppala
Year: September 24, 2021
Format: PDF
File size: PDF 21 MB
Language: English
Year: September 24, 2021
Format: PDF
File size: PDF 21 MB
Language: English
Overcome advanced challenges in building end-to-end ML solutions by leveraging the capabilities of Amazon SageMaker for developing and integrating ML models into productionKey FeaturesLearn best practices for all phases of building machine learning solutions - from data preparation to monitoring models in productionAutomate end-to-end machine learning workflows with Amazon SageMaker and related AWSDesign, architect, and operate machine learning workloads in the AWS CloudBook DescriptionAmazon SageMaker is a fully managed AWS service that provides the ability to build, train, deploy, and monitor machine learning models. The book begins with a high-level overview of Amazon SageMaker capabilities that map to the various phases of the machine learning process to help set the right foundation. You'll learn efficient tactics to address data science challenges such as processing data at scale, data preparation, connecting to big data pipelines, identifying data bias, running A B tests, and model explainability using Amazon SageMaker. As you advance, you'll understand how you can tackle the challenge of training at scale, including how to use large data sets while saving costs, monitoring training resources to identify bottlenecks, speeding up long training jobs, and tracking multiple models trained for a common goal. Moving ahead, you'll find out how you can integrate Amazon SageMaker with other AWS to build reliable, cost-optimized, and automated machine learning applications. In addition to this, you'll build ML pipelines integrated with MLOps principles and apply best practices to build secure and performant solutions.By the end of the book, you'll confidently be able to apply Amazon SageMaker's wide range of capabilities to the full spectrum of machine learning workflows.What you will learnPerform data bias detection with AWS Data Wrangler and SageMaker ClarifySpeed up data processing with SageMaker Feature StoreOvercome labeling bias with SageMaker Ground TruthImprove training time with the monitoring and profiling capabilities of SageMaker DebuggerAddress the challenge of model deployment automation with CI CD using the SageMaker model registryExplore SageMaker Neo for model optimizationImplement data and model quality monitoring with Amazon Model MonitorImprove training time and reduce costs with SageMaker data and model parallelismWho this book is forThis book is for expert data scientists responsible for building machine learning applications using Amazon SageMaker. Working knowledge of Amazon SageMaker, machine learning, deep learning, and experience using Jupyter Notebooks and Python is expected. Basic knowledge of AWS related to data, security, and monitoring will help you make the most of the book.Table of ContentsAmazon SageMaker OverviewData Science EnvironmentsData Labeling with Amazon SageMaker Ground TruthData Preparation at Scale Using Amazon SageMaker Data Wrangler and ProcessingCentralized Feature Repository with Amazon SageMaker Feature StoreTraining and Tuning at ScaleProfile Training Jobs with Amazon SageMaker DebuggerManaging Models at Scale Using a Model RegistryUpdating Production Models Using Amazon SageMaker Endpoint Production VariantsOptimizing Model Hosting and Inference Costs and u003c
