
BOOKS - PROGRAMMING - Collaborative Filtering Recommender Systems

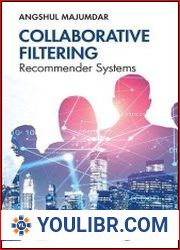
US $5.74

329447

329447
Collaborative Filtering Recommender Systems
Author: Angshul Majumdar
Year: 2025
Number of pages: 142
Format: PDF
File size: 14.2 MB
Language: ENG
Year: 2025
Number of pages: 142
Format: PDF
File size: 14.2 MB
Language: ENG
This book dives into the inner workings of recommender systems, those ubiquitous technologies that shape our online experiences. From Netflix show suggestions to personalized product recommendations on Amazon or the endless stream of curated YouTube videos, these systems power the choices we see every day. Collaborative filtering reigns supreme as the dominant approach behind recommender systems. This book offers a comprehensive exploration of this topic, starting with memory-based techniques. These methods, known for their ease of understanding and implementation, provide a solid foundation for understanding collaborative filtering. As you progress, you’ll delve into latent factor models, the abstract and mathematical engines driving modern recommender systems. The journey continues with exploring the concepts of metadata and diversity. You’ll discover how metadata, the additional information gathered by the system, can be harnessed to refine recommendations. Additionally, the book delves into techniques for promoting diversity, ensuring a well-balanced selection of recommendations. Finally, the book concludes with a discussion of cutting-edge Deep Learning models used in recommender systems. Recommender systems, powered by sophisticated algorithms and Machine Learning techniques, analyze user behavior, preferences, and past consumption patterns to identify patterns and predict future choices. These systems act as digital surrogates for the trusted friends, family, and experts who traditionally guided consumer decisions in the pre-internet era. The book assumes a basic understanding of linear algebra and optimization but requires no prior knowledge of Machine Learning or programming.
