
BOOKS - PROGRAMMING - Machine Learning in Pure Mathematics and Theoretical Physics

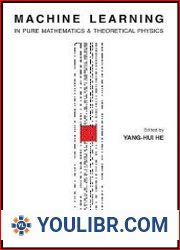
US $6.73

500936

500936
Machine Learning in Pure Mathematics and Theoretical Physics
Author: Yang-Hui He
Year: 2023
Format: PDF
File size: 32.4 MB
Language: ENG
Year: 2023
Format: PDF
File size: 32.4 MB
Language: ENG
The juxtaposition of "Machine Learning" and "pure mathematics and theoretical physics" may first appear as contradictory in terms. The rigours of proofs and derivations in the latter seem to reside in a different world from the randomness of data and statistics in the former. Yet, an often under-appreciated component of mathematical discovery, typically not presented in a final draft, is experimentation: both with ideas and with mathematical data. Think of the teenage Gauss, who conjectured the Prime Number Theorem by plotting the prime-counting function, many decades before complex analysis was formalized to offer a proof. Can modern technology in part mimic Gauss's intuition? The past five years saw an explosion of activity in using AI to assist the human mind in uncovering new mathematics: finding patterns, accelerating computations, and raising conjectures via the Machine Learning of pure, noiseless data. The aim of this book, a first of its kind, is to collect research and survey articles from experts in this emerging dialogue between theoretical mathematics and Machine Learning. It does not dwell on the well-known multitude of mathematical techniques in Deep Learning, but focuses on the reverse relationship: how Machine Learning helps with mathematics. Taking a panoramic approach, the topics range from combinatorics to number theory, and from geometry to quantum field theory and string theory. Aimed at PhD students as well as seasoned researchers, each self-contained chapter offers a glimpse of an exciting future of this symbiosis.
