
BOOKS - Data-Driven Clinical Decision-Making Using Deep Learning in Imaging

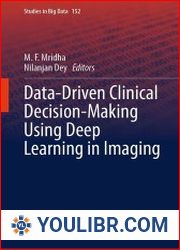
US $5.78

101918

101918
Data-Driven Clinical Decision-Making Using Deep Learning in Imaging
Author: M. F. Mridha, Nilanjan Dey
Year: 2024
Number of pages: 277
Format: PDF | EPUB
File size: 42.7 MB
Language: ENG
Year: 2024
Number of pages: 277
Format: PDF | EPUB
File size: 42.7 MB
Language: ENG
This book explores cutting-edge medical imaging advancements and their applications in clinical decision-making. The book contains various topics, methodologies, and applications, providing readers with a comprehensive understanding of the field's current state and prospects. It begins with exploring domain adaptation in medical imaging and evaluating the effectiveness of transfer learning to overcome challenges associated with limited labeled data. Machine Learning (ML) and Deep Learning (DL) have become indispensable for medical image analysis due to their capacity to extract meaningful information from large and complex medical image datasets. Currently, medical image datasets are increasing rapidly in size and complexity. Additionally, these algorithms are capable of processing and analyzing enormous amounts of data much more quickly and precisely than manual methods. However, it is challenging to determine which of these two approaches yields more accurate results. To analyze the efficacy of the ML strategy, seven distinct Machine Learning algorithms, including K-Nearest Neighbor (KNN), Logistic Regression (LR), Support Vector Machine (SVM), Decision Tree (DT), Naive Bayes (NB), RandomForest, and AdaBoost, are employed, followed by the best classifier being chosen using GridSearchCV. On the other hand, Convolutional Neural Network (CNN) dependent DL frameworks are designed for running an analysis of the Deep Learning approach.
